09.04.2020
5 minutes of reading
Thesis research conducted at IFPEN [1] developed tools that can be used to describe biogenic carbon flows on the basis of dynamic models rather than conventional static models. The analysis then focused on the differences in results and conclusions generated by this new approach, as well as its advantages in terms of climate forecasts.
Climate change: incorporating the time factor into the evaluation of carbon flows
Low-carbon strategies promote the use of renewable energy sources and biomaterials derived, among others, from dedicated forestry and agricultural biomass, as well as residual biomass. They are designed to achieve carbon neutrality, which consists in obtaining a perfect balance between the amount of CO2 emitted and the amount captured.
Climate change caused by human activities is also assessed using dedicated methodologies, such as life cycle analysis (LCA) or the carbon footprint, for example.
However, the models underlying these methodologies are static, since they only represent systems in steady-state conditions . For example, the life cycle inventory is based on a simplified aggregation of all the flows from various units of the process analyzed and, consequently, does not take into account the variability of carbon flows (fossil and biogenic1) over time.
The climate neutrality hypothesis relating to biogenic carbon emissions (Cbio) is thus justified by the fact that biomass carbon emission and sequestration processes are supposed to take place “at the same time”. Incorporating the time dimension, whereby Cbio flows are distributed over time, calls into question this climate neutrality.
Dynamic models applicable to all demand models
The new modeling framework proposed within the thesis research conducted at IFPEN takes into account:
a) upstream (production), non-linear models of biomass growth [2] and soil organic carbon (SOC) sequestration associated with the use of land, including crop management practices [3],
b) downstream models, for specific end-of-life options (waste and residue management), that may potentially delay emissions.
The dynamic models developed have been designed to be combined with the results of any demand model - giving technical flows that govern the quantity of biomass supplied/used in a studied system - in order to be able to produce full dynamic carbon inventories (fossil + biogenic).
For example, research studying the consequences of public policies for the energy transition (diagram below) combined a partial-equilibrium economic model of the French energy sector (Demand model) with a dynamic model for forestry biomass growth (Cbio model) to evaluate prospective bioenergy scenarios [4].
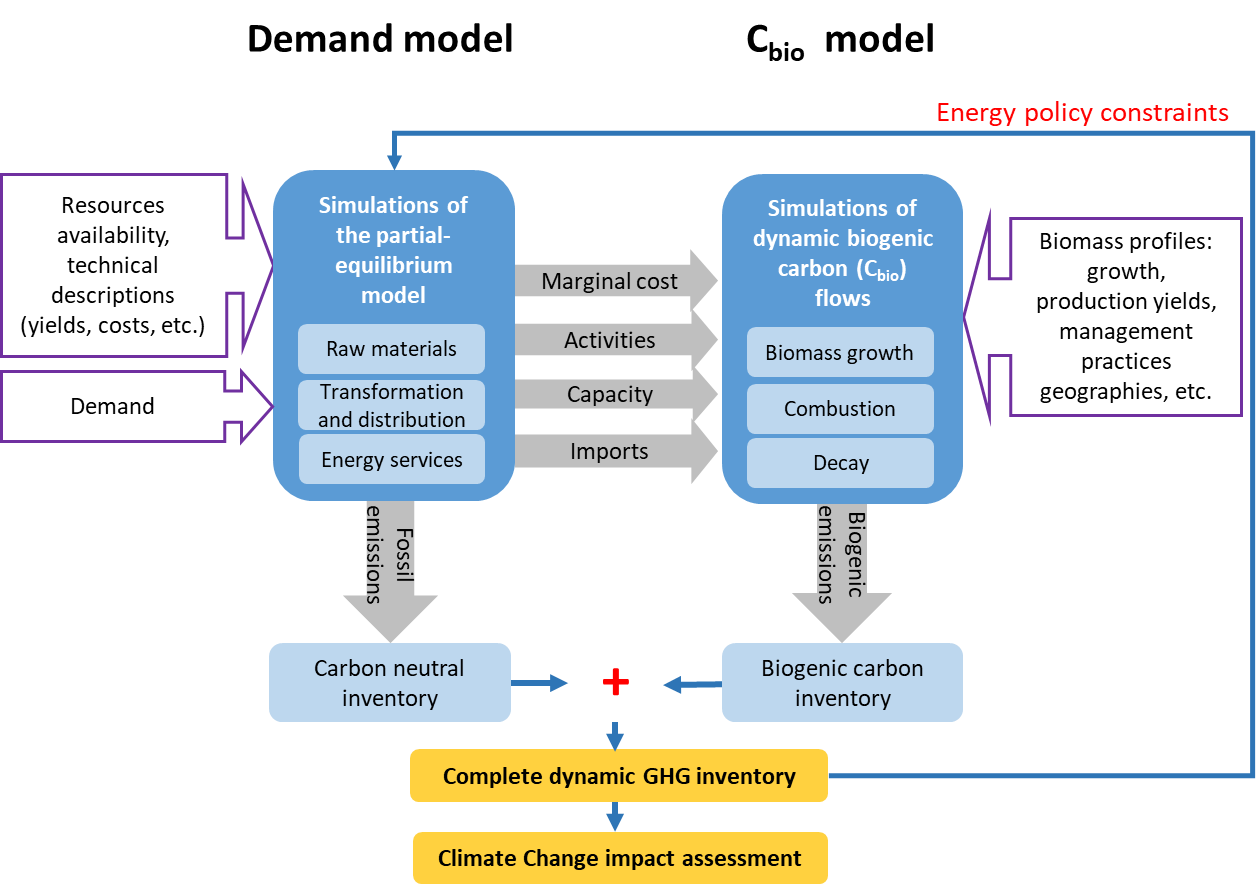
A methodological advance for more accurate predictions
The results showed that this new Cbio sequestration and SOC dynamics modeling approach provided a more accurate representation of Cbio flows and that its incorporation into climate change calculations had a significant impact on forecasts.
Moreover, this new tool has made it possible to underline the impact on climate change of the biomass used (particularly crop rotation durations), the export rates for residues for energy usages and the point at which the first sequestration flow takes place (before or after biomass use) [5].
This new dynamic approach may be further refined to take into account site or case-specific contexts.
The research conducted already makes it possible to take better account of biogenic carbon and helps to optimize LCA. A methodological advance of this type is particularly useful to help define initiatives aimed at tackling climate change.
1 Carbon from the terrestrial biosphere (around 20% in living biomass, 80% in dead organic biomass contained in forest litter and soils)
2 Prospective energy model https://fr.wikipedia.org/wiki/MARKAL#TIMES
References :
[1] Albers A, 2019. Prise en compte de la dimension temporelle dans l'évaluation environnementale des produits de la biomasse : Modélisation dynamique du carbone. Thèse soutenue le 16 décembre 2019 à Montpellier SupAgro.
[2] Albers A, Collet P, Benoist A, Hélias A (2019). Data and non-linear models for the estimation of biomass growth and carbon fixation in managed forests. Data in brief 23, 103841.
[3] Albers A., Avadi A., Benoist A., Collet P., A. Hélias (2019c). Modelling dynamic soil organic carbon flows of annual and perennial energy crops to inform energy-transport policy scenarios in France. Science of The Total Environment, 135278.
[4] Albers A, Collet P, Lorne D, Benoist A, Hélias A (2019a). Coupling partial-equilibrium model and dynamic biogenic carbon inventories for energy policy assessment. Applied Energy 239: 316-330.
[5] Albers A, Collet P, Benoist A, Hélias A (2019d). Back to the future: Dynamic full carbon accounting applied to prospective bioenergy scenarios. Int. J. Life Cycle Assess, 1-17.
Scientific contact : pierre.collet@ifpen.fr