Ensuring compatibility between polymers and fluids is essential in numerous industrial sectors: in the automotive sector, for example, the resistance of materials used in the fuel supply system is a vital consideration. For the components concerned, experimental methods are used to anticipate potential degradation of initial properties. However, these methods remain extremely time-consuming. A more appropriate alternative approach has emerged: use existing data, supplementing it if necessary, to extract information via methods borrowed from data science [1,2].
Cheminformatics lies at the interface of several scientific fields and involves using computer and informational techniques to solve problems related to chemistry. One aspect is the use of artificial intelligence to predict usage properties. In addition to data quality, one of the keys to success resides in the representation of the complex fluids considered, such as the thousands of compounds present in fuels.
Despite constant improvements in analysis techniques, accurately identifying each component of these complex fluids appears to be an insurmountable task. Thanks to two-dimensional chromatography (GC×GC), it is nevertheless possible to quantify the chemical families present and obtain distributions based on the number of carbon atoms. A representative molecule is associated with each component of the distribution: for example, a jet fuela can be structurally represented by a mixture of around one hundred compounds [1].
To ensure it can be interpreted by machine learning algorithms, each of these molecules must be encoded by molecular descriptors. Since these are numerous, the choice was made to use simple descriptors derived from counts of the functional groups present. A descriptor vector encoding the fluid (figure 1) is then obtained thanks to a linear mixing rule applied to the descriptors, the components and their respective fractions.
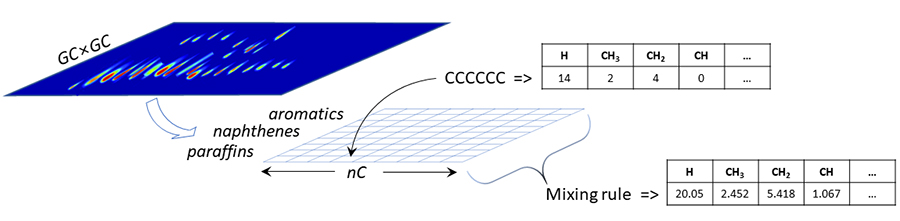
This methodology has been used to model the quantity of fluid penetrating a polymer when the two come into contact with each other. Different polymer/fluid combinations have thus been studied, databases created and machine learning algorithms applied.
The models obtained make it possible to instantly anticipate the impact of adding biofuels to gasolines, or the use of alternative jet fuels on the properties of various polymers (figure 2).
Such a modeling approach helps to significantly reduce the time required to quantify polymer/fluid compatibility.
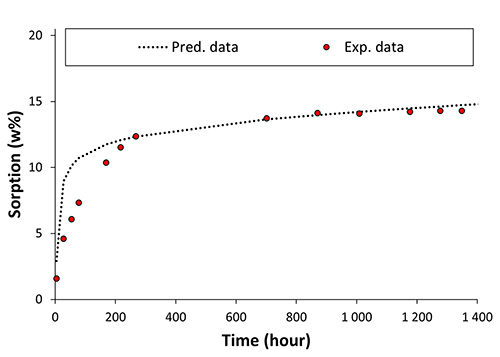
In the context of the representation of complex fluids, at least two types of developments can be envisaged:
- integration of the in silico generation of structures [3] to obtain an even more detailed characterization;
- the application of this modeling methodology to the modeling of other phenomena, such as fluid aging, for example.
a- or kerosene
b- Material used in fuel circuits: tanks, pipes, gaskets
References:
- B. Creton, B. Veyrat, M.-H. Klopffer, Fuel sorption into polymers: experimental and machine learning studies, Fluid Phase Equilibria 2022, 556, 113403.
>> DOI: 10.1016/j.fluid.2022.113403
- N. Villanueva, B. Flaconnèche, B. Creton, Prediction of alternative gasoline sorption in a semicrystalline poly(ethylene), ACS Combinatorial Science 2015, 17(10), 631-640.
>> DOI: 10.1021/acscombsci.5b00094
- C. Hall, B. Creton, B. Rauch, U. Bauder, M. Aigner, Probabilistic Mean Quantitative Structure Property Relationship modelling of Jet Fuels Properties, Energy & Fuels, 2022, 36(1), 463-479.
>> DOI: 10.1021/acs.energyfuels.1c03334
Scientific contact: benoit.creton@ifpen.fr
You may also be interested in
Cheminformatics and its descriptors: application to polymer/fluid compatibility
Ensuring compatibility between polymers and fluids is essential in numerous industrial sectors: in the automotive sector, for example, the resistance of materials used in the fuel supply system is a vital consideration.
Review of a Chair on “thermodynamics for biomass fuels”
Unlike hydrocarbons of fossil origin, the molecules derived from biomass are polar, due to the heteroatoms they contain. This difference on a molecular scale induces a more complex macroscopic behavior that must be taken into account when designing the processes where such mixtures are encountered.