Today, characterization of geological reservoirs, a long-standing theme in petroleum exploration, becomes a base of interest for a variety of applications, such as CO2 and hydrogen storage as well as geothermal energy. In recent years, the combined use of 3D microtomography (or micro-CT1) imaging and advanced simulation techniques has allowed the emergence of a digital approach to computing the petrophysical properties of reservoir rocks (Digital Rock Physics). This represents a real complement - and in some cases an alternative - to traditional laboratory measurements.
To this end, IFPEN has carried out an unprecedented tomographic image acquisition campaign, using the PSICHE beamline of the SOLEIL synchrotron, on a hundred rock samples (1 cm in diameter and 2 cm in length). The voluminous and exclusive database2 of 3D images thus generated was then used to predict petrophysical properties.
Usually, numerical methods used to compute physical properties from 3D images3 are very time-consuming and often limited by the resolution of these images. For this work, researchers at IFPEN have deployed an alternative method based on Deep Learning, capable of fully exploiting the large volume of acquired data [1]. This ambitious approach allowed to reconcile the huge amount of data with neural architectures, drawing on 3D convolutional neural networks4. It required the use of large parallel calculation resources, such as graphic processors5, accessible on the Jean-Zay supercomputer of GENCI6.
These calculations led to a good prediction performance concerning the permeability of the studied rocks (figure), thereby demonstrating the potential of deep learning methods in the field.
Click on the picture to enlarge
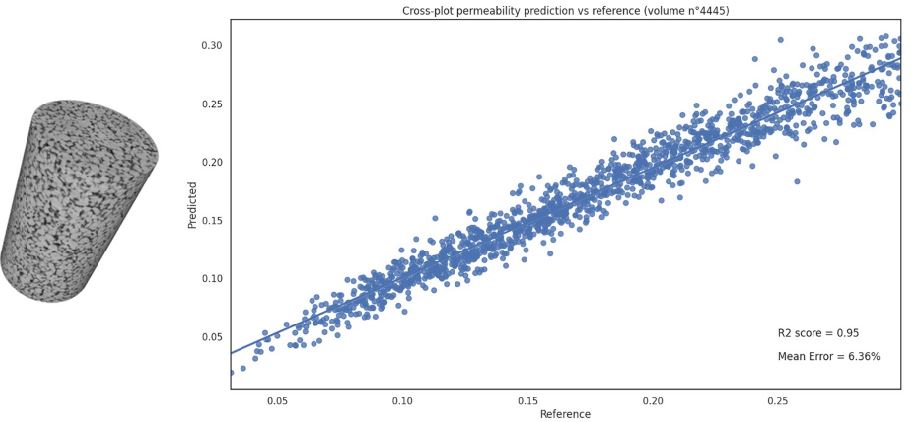
However, questions remain concerning the generalization of the prediction quality to other rocks and research is underway to confirm its relevance for this use.
A first aspect of this research consists in using learning methods further upstream, for example to deduce properties of interest, at the voxel scale. The ambition is to predict velocity fields at all points of the volume to enable more accurate permeability calculations.
The other aspect concerns the use of specific neural architectures to improve the resolution of the images acquired, a major challenge for the implementation of learning models.
1- Micro Computed Tomography
2- 32 Go memory for each plug due to digitalization with a resolution of 5.8 µm
3- Such as Porous Network Modeling (PNM) or the Lattice Boltzmann Method (LBM)
4- Multilayer neural networks with a connection architecture inspired by that of the visual cortex of mammals
5- Graphic Processor Unit (GPU)
6- National High-Performance Computing Equipment
Publication:
[1] S. Youssef, G. Batot, F. Cokelaer, S. Desroziers et M. Feraille, On the Performance of Deep Learning Methods for Rock Property Prediction from 3D Micro-CT Images, currently being drafted
Scientific contact: souhail.youssef@ifpen.fr