Inside porous materials, physico-chemical phenomena such as matter transport, catalytic reactions and capillary effects are strongly influenced by the geometry of the pore networks, i.e., the degree of porosity, the distribution of pore sizes and their connectivity. These characteristics are usually obtained via macroscopic models applied to experimental characterization results. Additionally, a reliable estimation of these properties on a smaller scale can come from so-called microscopic approaches, via statistical mechanics.
IFPEN and Saint Gobain Research Provence decided to tackle the problem differently, by exploring a new numerical approach. This approach directly combines realistic numerical modeling of microstructures with a novel simulation framework of gas physisorption from a morphological point of view.
The approach was hinged around three components:
- random models to realistically represent complex microstructures;
- mathematical morphology to reproduce the various physical processes involved in physisorption [1];
- and deep learning to accelerate simulation results [2].
Since the new approach consists in artificially reproducing physical phenomena using mathematical morphology operators, it paves the way for physisorption simulations, with results directly comparable to experimental results. The calculation of these operations can be estimated via deep learning, using convolutional neural networks , leading to a considerable reduction in calculation time.
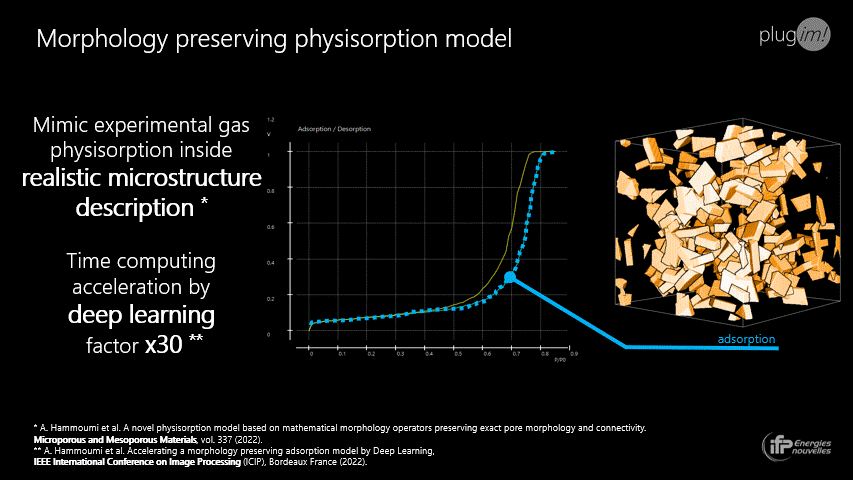
This original methodology was successfully applied to ordered structures such as SBA-15 and KIT-5 aluminas, leading to numerical isotherms close to experimental isotherms (figure). Comparable results were obtained for more complex aluminas, with controlled multi-scale porosity specifically synthesized for this research [3].
Extending the approach to other experimental techniques for the characterization of porous materials is the next step in the continuation of this research, the ultimate objective of which is to obtain innovative materials designed directly on the basis of the targeted usage properties.
References:
-
Hammoumi A., Moreaud M., Jeulin D., Jolimaitre E., Chevalier T., Sorbier L., Klotz M., Novikov A., A novel physisorption model based on mathematical morphology operators preserving exact pore morphology and connectivity, Microporous and Mesoporous Materials, (2022).
>> https://doi.org/10.1016/j.micromeso.2022.111847
-
Hammoumi A., Moreaud M., Jolimaitre E., Chevalier T., Klotz M., Novikov A. Accelerating a Morphology-Preserving Adsorption Model by Deep Learning, 2022 IEEE International Conference on Image Processing (ICIP), (2022).
>> https://doi.org/10.1109/ICIP46576.2022.9897406
-
Hammoumi A., Analysis-Driven Design of Digital Multi-scale Microstructures of Materials. PhD thesis, Université Paris-Saclay, (2022).
>> https://www.theses.fr/2022UPASG083
Scientific Contacts: adam.hammoumi@ifpen.fr ; Maxime Moreaud
You may also be interested in
Diffusion in catalysts: an often tortuous path!
In catalytic processes, an active phase is necessary to accelerate the transformation of the molecules in the fluid treated. Most of the time, this catalytic agent is placed on a porous support with a large internal surface area, making it possible to host a large number of active sites within a small volume...
Numerical design based on the analysis of multi-scale porous material microstructures
The design of high-quality porous materials is a major challenge for the energy efficiency of industrial processes in the fields of catalysis and biocatalysis and separation and purification operations. For such applications, these materials derive their properties of interest from their specific microstructure, incorporating a large quantity of empty spaces that are organized and connected on a nanometric scale. IFPEN and Saint Gobain Research Provence (SGRP) joined forces to acquire a tool that will ultimately facilitate the development of porous materials optimized for given usages.