Over the last decade, deep learning applied to image analysis has rapidly developed in scope to cover numerous fields. However, its potential remains underexploited in geology, despite the fact that it is a discipline that relies to a large extent on visual interpretation. To contribute to the digital transformation of industries related to the underground environment, researchers at IFPEN have implemented deep learning in three “profession-specific contexts”, each involving different types of geological images.
The first application [1] is an image classification of macroscopic rock samples with convolutional neural networks1.
Researcher began by implementing and comparing different neural architectures and learning strategies considered in the scientific literature as references for image analysis. This enabled them to construct a first effective prediction tool.
They then adopted an original approach mirroring that of a geologist, based on a cascade-neural-network model tree. This method facilitates the integration of geological knowledge in the statistic model and offers an increased capacity to explain predictions. It also complements the first model by compensating for certain errors (Figure 1).
Click on the picture to enlarge
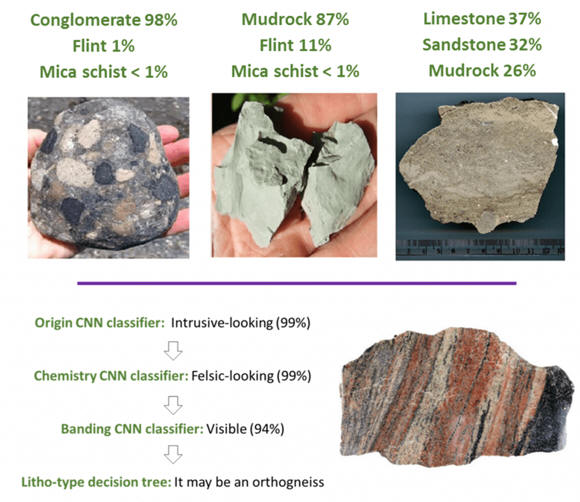
Top: direct classification. The three most likely classes according to the neural network are displayed on each image. The first two images are archetypal and probabilities clearly point to a single class. However, the third image is more ambiguous and the probabilities reflect the uncertainties a human geologist would be faced with.
Bottom: lithological classification combining recognition of the petrological characteristics and a decision tree.
In the second application [2], detection algorithms were employed to define and categorize microfossils on digitized images of thin rock sections.
Several deep learning methods for the detection of objects, based on the state of the art, were compared for a first set of data limited to 15 annotated images. The results for 130 other images of thin sections were qualitatively evaluated by expert sedimentologists, with quantitative measurements of precisions and inference times2. This work constitutes a proof of concept for the automated identification of paleofauna, since models demonstrated a good capacity to detect and categorize microfossils (Figure 2). However, differences in precision and performance were highlighted, leading to recommendations being made for their use in similar projects.
Click on the picture to enlarge
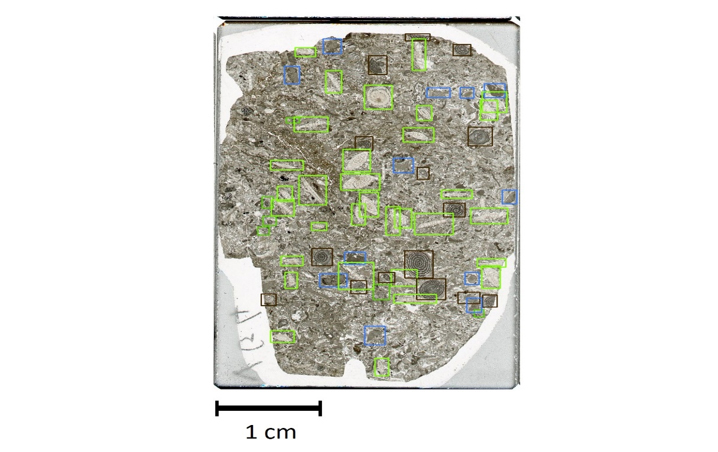
The third application [3] is an automated lithological characterization of geological core images. The data considered come from an IODP (International Ocean Drilling Program) drilling campaign in the Corinthian Gulf. They were gathered at 3 different sites and were interpreted by an expert in 17 facies associations. In this work, different challenges and potential solutions were highlighted in order to manage situations in which little training data is available. In particular, transfer learning3, as well as hyperparameter calibration4, prove to be crucial for the development of an effective predictive system.
This body of research highlights the potential of deep learning methods to obtain relevant geological information from images, while underlining the importance of adapting them to the specific applications concerned.
1- Multilayer neural networks with a connection architecture inspired by that of the visual cortex of mammals.
2- Deduction process based on implicit information
3- Consisting of applying knowledge obtained by carrying out a task in order to solve a different problem but with certain similarities.
4- In machine learning, a hyperparameter is a variable the value of which is used to control the training process.
Publications:
[1] A. Bouziat, S. Desroziers, M. Feraille, J. Lecomte, R. Divies et F. Cokelaer, Deep Learning Applications to Unstructured Geological Data: From Rock Images Characterization to Scientific Literature Mining, First EAGE Digitalization Conference and Exhibition, Nov 2020, Volume 2020
>> https://doi.org/10.3997/2214-4609.202032047
[2] A. Koroko, A. Lechevallier, M. Feraille, J. Lecomte, A. Bouziat et S. Desroziers, Appraisal of several Deep Learning models for microfossil identification on thin section images, Second EAGE Workshop on Machine Learning, Mar 2021, Volume 202,
>> https://doi.org/10.3997/2214-4609.202132005
[3] A. Lechevallier, A. Bouziat et S. Desroziers, Assisted interpretation of core images with Deep Learning workflows: lessons learnt from a practical use case, Second EAGE Workshop on Machine Learning, Mar 2021, Volume 2021,
>> https://doi.org/10.3997/2214-4609.202132003
Scientific contacts: antoine.bouziat@ifpen.fr and sylvain.desroziers@ifpen.fr